Unlocking the Future: Mastering Machine Learning Labeling Services
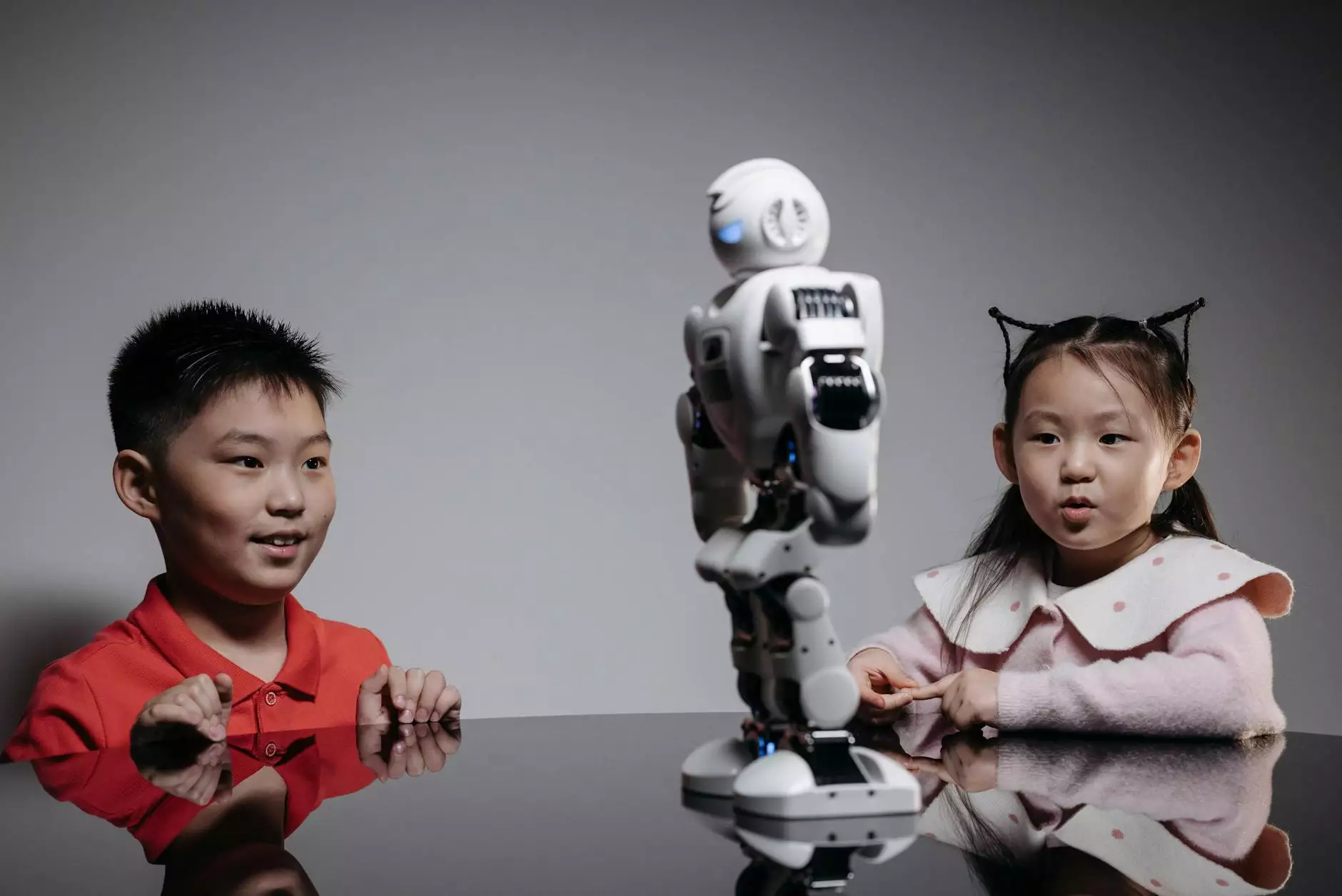
Machine learning is revolutionizing industries by enabling computers to learn from data and make decisions. However, for these systems to function effectively, they require accurately labeled datasets. This is where a machine learning labeling service plays a crucial role, serving as the backbone of any successful AI initiative.
Understanding Machine Learning Labeling Services
A machine learning labeling service involves the process of annotating data so that a machine learning model can interpret it correctly. This includes various types of data, such as text, images, videos, and audio, which need precise labeling to train algorithms effectively.
The Importance of Data Labeling in Machine Learning
Data labeling is integral to the machine learning process, as it provides the context needed for algorithms to learn. Think of it as teaching a child to recognize objects—the child needs to know what to call each object before they can start identifying them. Without proper labeling, even the most sophisticated algorithms may fail to perform accurately. Here are some reasons why data labeling is essential:
- Increases Accuracy: Labeled data improves the precision of machine learning models.
- Enhances Predictive Power: With accurate labels, the model can make better predictions.
- Aids in Understanding Data: Labeling acts as a tool for gaining insights from complex datasets.
Types of Machine Learning Labeling Services
Machine learning labeling services can vary based on the data type and the complexity of the required labels. Below are the main categories of labeling services:
1. Image Annotation
For computer vision applications, accurate image annotation is pivotal. Services can include:
- Bounding Boxes: Framing objects within an image.
- Semantic Segmentation: Classifying each pixel in an image.
- Keypoint Annotation: Marking significant points in an image.
2. Text Annotation
Text data requires a different approach, often involving:
- Entity Recognition: Identifying and classifying key elements in text.
- Sentiment Analysis: Determining the underlying sentiment behind text.
- Intent Labeling: Understanding the intent behind user queries.
3. Audio Annotation
In audio processing, labeling may involve:
- Transcription: Converting spoken words into text.
- Speaker Recognition: Identifying different speakers in a recording.
- Emotion Detection: Understanding emotional tones in speech.
How to Choose the Right Machine Learning Labeling Service
Choosing the correct labeling service can significantly impact your project's success. Here are essential factors to consider:
1. Quality Assurance
Look for services that have stringent quality assurance protocols in place. High-quality labeling ensures your AI systems learn accurately.
2. Scalability
Your labeling service should be able to scale according to your project size. This is particularly important if you anticipate changes in data volume.
3. Expertise in Domain
A labeling service that understands your industry can provide more relevant and context-aware annotations.
4. Technology Utilization
Utilization of modern AI tools for pre-labeling can enhance efficiency and reduce costs.
The Benefits of Machine Learning Labeling Services for Businesses
Implementing machine learning labeling services brings numerous benefits to businesses across various industries:
1. Improved Model Performance
High-quality labels allow machine learning models to perform at their peak, leading to improved results in real-world applications.
2. Cost-Effectiveness
By outsourcing labeling tasks, companies can save on the cost of hiring full-time staff dedicated to this process.
3. Time Efficiency
Automating the labeling process can drastically reduce the time required to prepare datasets, allowing teams to focus on model development and deployment.
4. Enhanced Competitive Advantage
Companies with better-informed models can outpace competitors, driving innovation and efficiency within their sectors.
Case Studies: Success Through Machine Learning Labeling Services
Case Study 1: Retail Industry
In a notable case within the retail sector, a major e-commerce platform utilized an image annotation machine learning labeling service to enhance its product recognition models. By implementing precision image labeling, the platform saw a 25% increase in search accuracy, leading to improved customer satisfaction and higher conversion rates.
Case Study 2: Healthcare Sector
In healthcare, machine learning solutions are emerging to analyze medical images. A hospital partnered with a labeling service specializing in medical image annotation. This collaboration enabled the hospital to develop diagnostic tools that support early detection of diseases, significantly impacting patient outcomes.
Future Trends in Machine Learning Labeling Services
The field of machine learning labeling services continues to evolve with advancements in technology. Here are some trends to watch:
1. Automation and AI in Labeling
Expect greater use of AI and automation tools to assist with data labeling, reducing human effort while maintaining quality standards.
2. Crowdsourcing for Data Labeling
Crowdsourcing is gaining popularity for its ability to rapidly label vast datasets, harnessing diverse human intelligence for nuanced understanding.
3. Increased Focus on Ethical AI
The future will see a rising demand for ethical considerations in labeling, ensuring that datasets are free from biases and reflect real-world diversity.
Conclusion: Empower Your Business with Machine Learning Labeling Services
As the reliance on machine learning continues to grow, so does the importance of effective labeling services. By embracing a machine learning labeling service, businesses can unlock the full potential of their data, driving innovation and achieving their goals with greater efficiency. Explore the possibilities today and take your first step toward a future powered by intelligent, accurately-labeled data.